Right now, will acquire about the job of forecasts in nimble information science. The intelligent reports uncover various parts of information. Forecasts structure the fourth layer of nimble dash.
When making expectations, we generally allude to the past information and use them as derivations for future emphasess. Right now, we progress information from cluster handling of recorded information to continuous information about what's to come.
The job of forecasts incorporates the accompanying −
- Expectations help in determining. A few gauges depend on factual induction. A portion of the forecasts depend on assessments of savants.
- Factual induction are associated with forecasts of numerous types.
- Now and again figures are exact, while once in a while estimates are mistaken.
Predictive Analytics
Prescient investigation incorporates an assortment of measurable procedures from prescient displaying, AI and information mining which break down present and chronicled realities to make forecasts about future and obscure occasions.
Prescient examination requires preparing information. Prepared information incorporates autonomous and subordinate highlights. Subordinate highlights are the qualities a client is attempting to foresee. Free highlights are highlights portraying the things we need to anticipate dependent on subordinate highlights.
The investigation of highlights is called include building; this is essential to making expectations. Information perception and exploratory information examination are portions of highlight designing; these structure the center of Agile information science.
Making Predictions
There are two different ways of making forecasts in dexterous information science −
- Relapse
- Characterization
Building a relapse or a characterization totally relies upon business necessities and its investigation. Expectation of constant variable prompts relapse model and forecast of unmitigated factors prompts characterization model.
Regression
Relapse considers models that involve highlights and accordingly, produces a numeric yield.
Classification
Arrangement takes the information and produces a straight out characterization.
Note − The model dataset that characterizes contribution to measurable expectation and that empowers the machine to learn is designated "preparing information".
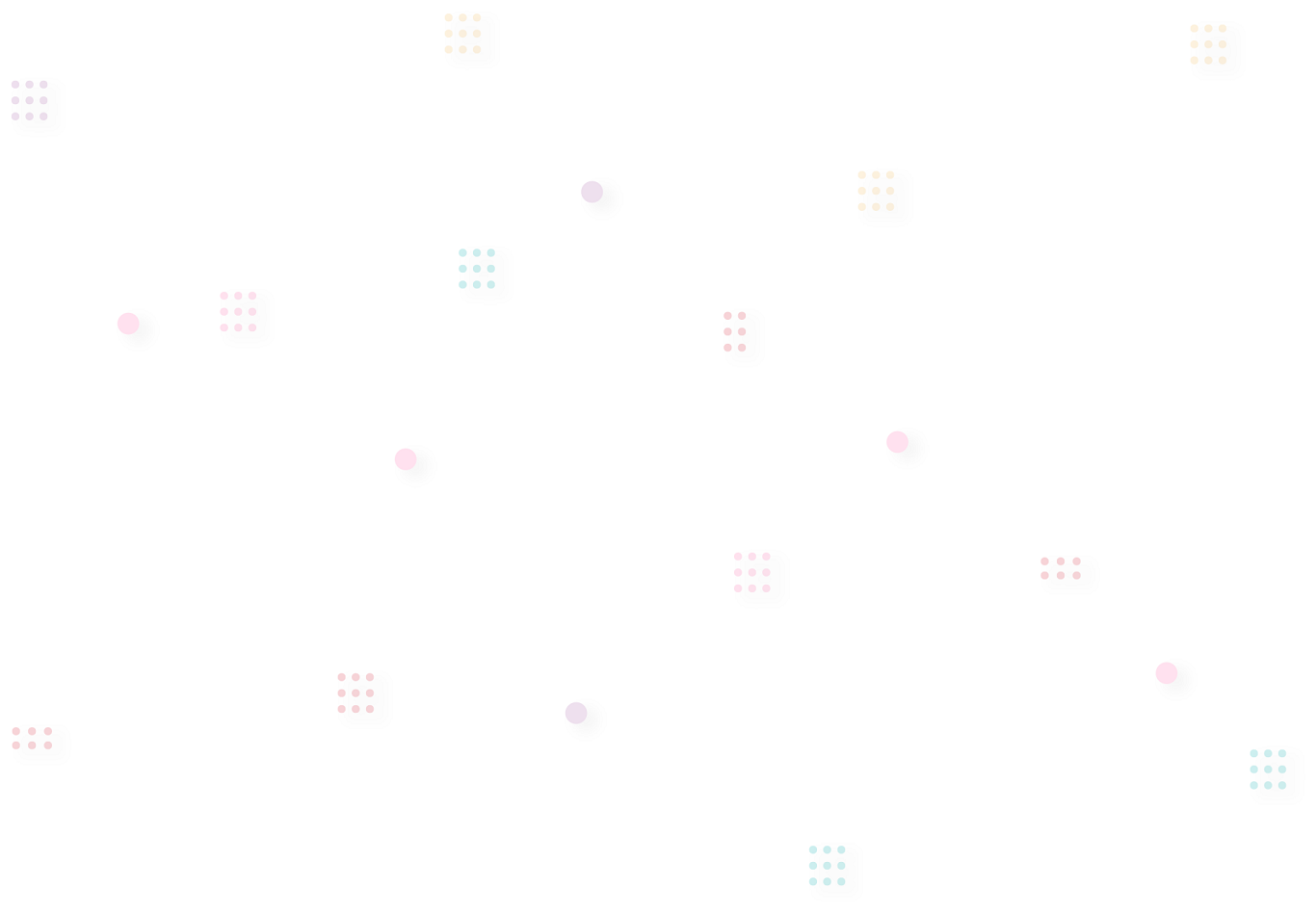